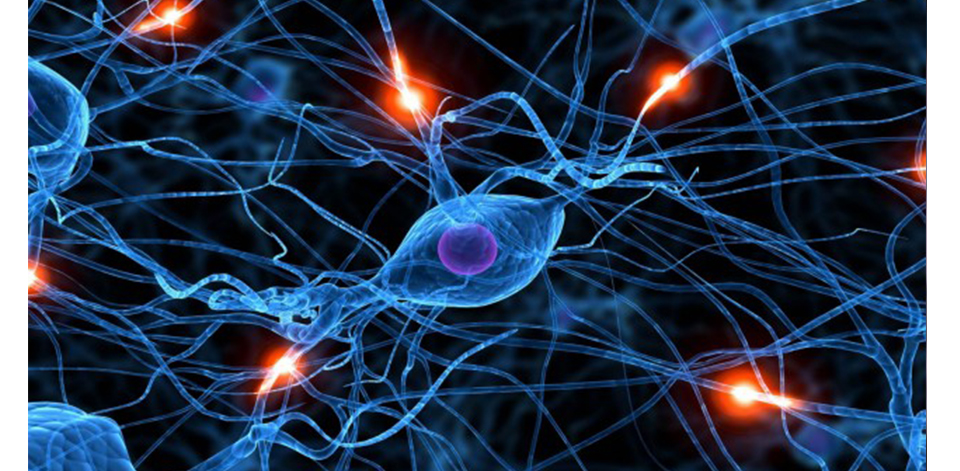
What is AI, Machine Learning & Deep Learning?
Feb 09,2018Introduction to Artificial Intelligence
Artificial intelligence (AI) is quite the buzz word nowadays, and everyone is talking about it. All significant think-tanks, consultancy firms, and other prominent corporate houses are churning out articles and thoughts on AI. They are also investing in the field. From self-driven cars to SIRI to robots performing surgeries, AI is omnipresent. Concepts like Machine Learning (ML) and Deep learning have also gained considerable momentum. As a matter of fact, we talk about them in the same vein.
AI and dependent concepts of ML and Deep Learning
So, are these concepts the same? Can we use them interchangeably? The answer is no! AI, ML, and Deep learning are not the same things. In the light of this, a look at the table below will give us a basic understanding of the three. It will also shed light on the main difference between them.
Artificial Intelligence (AI) | Machine Learning (ML) | Deep Learning (DL) |
---|---|---|
Human Intelligence exhibited by machines | A subset of AI, training machines to learn to act intelligently | A technique for implementing ML using neural networks to solve problems intelligently |
Example: A computer being able to identify spam | Defining algorithms that enable computers to identify and predict spam emails based on a few established criteria | Using extensive data to learn seemingly unobvious patterns and correlations to identify, for example, facial recognition, handwriting recognition, etc |
By the same token, the following section seeks to talk about the three in detail.
• When machines start to make intelligent, human-like decisions, observations, and simulations, it is termed AI. With the correct inputs, machines may begin to mimic human cognitive functions. These functions include problem-solving capacity, learning from experience, and adapting to changes in the environment. In essence, AI is incorporating thinking ability into machines.
• When we train a machine to display intelligence without needing to code them explicitly, it is Machine Learning. Therefore, ML can mean an extension of AI or its application. Moreover, if the system has successfully learned from the previous data, it will predict the future projections better. There exists a clear bifurcation in the types of machine learning, i.e., supervised, semi-supervised and unsupervised machine learning.
• A subset of ML is Deep learning. It is a class of algorithms using which we train machines to learn to identify hidden patterns that remain buried under layers of data. Deep learning uses coding that mimics the working of our nervous system. This is called the neural network. The algorithms identify the most hidden data patterns, trends, correlations, etc. Furthermore, data scientists believe that more substantial the amount of data handled, larger will be the models. This means that more will be the number of simulations performed. As a result, this means more ‘neural connections,’ ultimately culminating into better algorithms, new insights, and ultimately, results.
The field of data science has turned to machines to process and interpret a large volume of data. The underlying premise is that machines can learn on their own from experience. Therefore, just as humans learn from experience, a machine does the same. The additional advantage is that the interpretations or analysis derived are free from human prejudice. Thus, machines can give a result (sometimes, even smarter than humans), but without any chances of contamination by an individual’s thought. Additionally, more and more machines can do repetitive jobs. While for humans, it means a respite from boredom, for machines, it amounts to more simulations and better results. Unlike humans, machines can be made to work long hours without needing to take a break.
Extensive reach of Artificial Intelligence
AI is everywhere. Each sector of the economy is now showing a promise to gain by these technological advancements. Currently, AI is entering education, medicine, finance, and economics. The list is extensive. While there are several pros, increased use of AI raises ethical questions. One of them is its ability to replace a human being. While machines have exhibited great potential in ‘giving a judgment like an intelligent thinking individual,’ a complete exclusion of human factor is not a comforting thought. Further, with the technology still developing in this field, is there any danger that we face from machines? By creating machines that are capable of ‘independent thinking,’ are humans at risk? We are yet to be in a position to answer this conclusively.
For the most part, it is now evident that AI is one of the most driving forces today. Also, more and more industries are relying on AI, especially on machine learning. This is done to derive meaningful insights into their data and perform complex tasks. The advantages of this technically superior application are many. By and large, machines have always eased the human burden and aided us in our endeavors. In any event, the future of AI is still unpredictable.
References:
- https://www.techrepublic.com/article/understanding-the-differences-between-ai-machine-learning-and-deep-learning/
- https://towardsdatascience.com/deep-learning-weekly-piece-the-differences-between-ai-ml-and-dl-b6a203b70698?gi=c58e0a42e8c6
- https://dzone.com/articles/the-differences-between-ai-and-ml
- https://en.wikipedia.org/wiki/Artificial_intelligence
- http://searchcio.techtarget.com/definition/AI
- https://www.techopedia.com/definition/190/artificial-intelligence-ai
- https://www.toptal.com/machine-learning/machine-learning-theory-an-introductory-primer